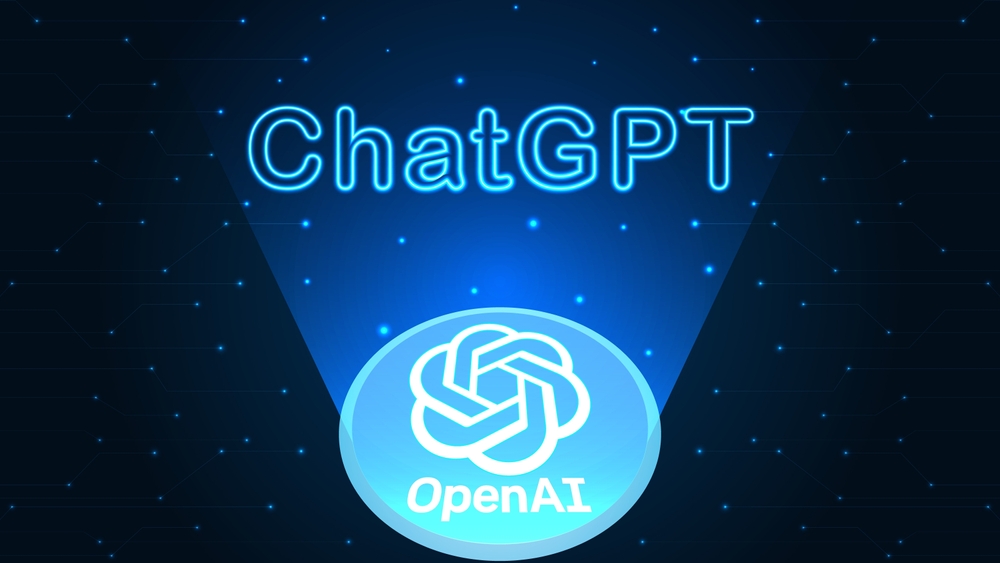
(Ebru-Omer/Shutterstock)
With its detailed and human-like written responses, ChatGPT has caught the world’s attention and spawned a meaningful discussion about how people should interact with this form of AI. ChatGPT is an upgrade in many ways from its predecessor, GPT-3.5, although it is still prone to making things up. But for production applications, AI developers may consider using ChatGPT in combination with other tools for a complete solution, experts say.
Developed by OpenAI and trained on Microsoft Azure, ChatGPT and GPT-3.5 are both conversational AI systems based on large language models, but there are important differences.
For starters, Generative Pretrained Transformer (GPT) 3.5 came before ChatGPT, and its neural network has more layers than ChatGPT. GPT-3.5 was developed as a general language model that can do multiple things, including translate language, summarize text, and answer questions. OpenAI has provided an API interface for GPT-3.5, which provides a more efficient way for developers to access its capabilities.
ChatGPT is based on GPT-3.5, but was developed specifically to be a chatbot (“conversational agent” is the preferred term of art in the industry). A limiting factor is that ChatGPT only sports a text interface; there is no API. ChatGPT was trained on a large set of conversational text and is better at holding up a conversation than GPT-3.5 and other generative models. It generates its responses more quickly than GPT-3.5, and its responses are perceived to be more accurate.
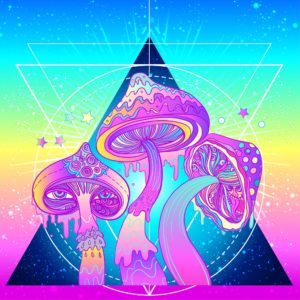
Far out, man: ChatGPT’s ‘hallucination rate’ has been cited as being between 15% and 21% (Gorbash-Varvara/Shutterstock)
However, both models have a tendency to make stuff up, or “hallucinating” things, as those in the industry call it. Various hallucination rates have been cited for ChatGPT between 15% and 21%. GPT-3.5’s hallucination rate, meanwhile, has been pegged from the low 20s to a high of 41%, so ChatGPT has shown improvement in that regard.
Despite the tendency to make things up (which is true with all language models), ChatGPT marks a significant improvement over the AI models that came before it, says Jiang Chen, founder and vice president of machine learning at Moveworks, a Silicon Valley firm that uses language models and other machine learning technologies in its AI conversational platform, which is used by companies in a variety of industries.
“ChatGPT does impress people, surprise people,” says Chen, who previously was a Google engineer who worked on the tech giant’s eponymous search engine. “The reasoning ability is something that probably surprised a lot of machine learning practitioners.”
Moveworks uses a variety of language models and other technologies to build custom AI systems for customers. It has been a big user of BERT, a language model open sourced several years ago by Google. The company uses GPT-3.5 and it’s already starting to use ChatGPT too.
However, when it comes to building a production conversational AI system, ChatGPT has its limitations, according to Chen. There are various tradeoffs at play in using these technologies to build a custom conversational AI system, Chen says, and it’s important to know where the lines are drawn to build a system that doesn’t provide the wrong answer, isn’t overly biased, and doesn’t make people wait too long.
ChatGPT is superior to BERT in terms of generating meaningful responses to questions, Chen says. Specifically, ChatGPT has more “reasoning” capability than BERT, which was designed to predict the next word in a sentence.
While ChatGPT and GPT-3.5 can provide compelling responses to questions, their closed, end-to-end nature prevents engineers like Chen from tinkering with them. That also presents a barrier when it comes to generating the answer on a custom corpus of words for a specific industry (retailers and manufacturers use different words than law firms and governments). The closed nature also raises difficulty when it comes to mitigating bias, he says.
BERT is small enough that it can be hosted by companies like Moveworks. The company has built a data pipeline that gathers data specific to a company and routes them into the BERT model for training. This work allows Moveworks to exert more control over the final conversational AI product, which is not something that’s possible with closed systems like GPT-3.5 and ChatGPT.
“Our machine learning stack is kind of layered,” Chen says. “We use BERT but we also use other machine learning algorithms, which allows us to incorporate customer-specific logic and customer-specific data into it.”
While the OpenAI models are much bigger and are trained on a much larger corpus of words, there’s no way to know if they’re the right words for a specific customer, Chen says.
“The [ChatGPT] model is pretrained to encode all the knowledge that is fed into it. It was not designed to do any specific task itself,” he says. “The reason it was able to speed up and achieve fast growth is because the architecture itself is actually simple. It’s layers and layers of the same stuff, so it’s kind of fused together. Because of that architecture, you know it learns something, but you don’t know where it encodes what information where. You don’t know what layers of neurons encode that specific information you want to inference it to, so it becomes more of a black box.”
ChatGPT may be going viral, but its usefulness as a production tool for conversational AI may be a bit overblown, in Chen’s opinion. Instead of going all-in with one specific model, a better approach is to leverage the strengths of multiple models, thereby driving better alignment with customers’ performance, accuracy, and bias expectations and the technologies’ underlying capabilities.
“Our strategy is using a different set of models in different places. You can use large models to teach your smaller models, and then the smaller models are much faster,” he says. “For example, if you wanted to do a segmented search, you want to use…some kind of BERT model, and then run that as some kind of vector search engine. ChatGPT is too big for that.”
While ChatGPT’s usefulness for real-world application may be relatively shallow at the moment, that doesn’t mean it’s not important. One of the lasting impacts that ChatGPT is likely to have is capturing practitioners’ attention and inspiring people to push the boundaries on what is possible in the future with conversational AI technology, Chen says.
“I do think it opens up a field,” he says. “I think going forward, when we open up the box, I think there will be a lot more interesting ways, interesting applications. It’s something we are excited about and are investing R&D on that.”
Related Items:
Microsoft Announces ChatGPT-powered Bing, Google CEO Declares ‘Code Red’
OpenAI’s New GPT-3.5 Chatbot Can Rhyme like Snoop Dogg
AI Is Coming for White-Collar Jobs, Too